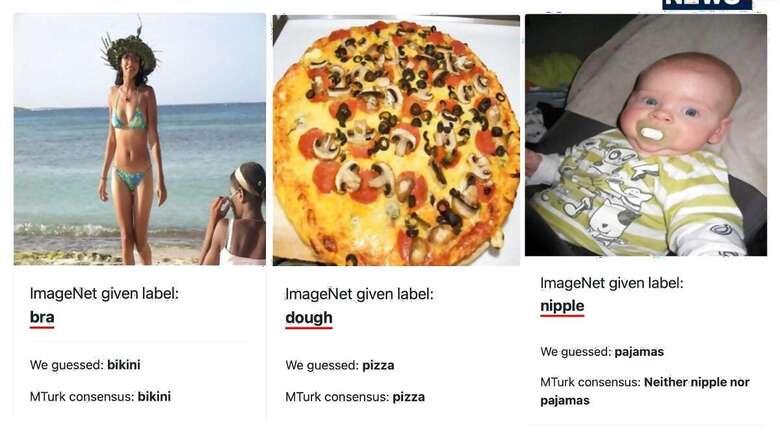
views
It turns out, AI has been getting quite a few things wrong all this while. A team of researchers led by MIT has discovered that a bit more than 3% of the data in the most used machine learning systems, has been labeled incorrectly. The researchers looked at 10 major machine learning data sets and find that 3.4% of the data available for the artificial intelligence machine learning systems has been mislabeled. There are multiple types of errors, including Amazon and IMDB reviews being incorrectly labeled as positive when they may actually be negative, and image-based tagging that may incorrectly identify the subject in the image. There are video based errors as well, such as a YouTube video being labeled as a church bell.
“We identify label errors in the test sets of 10 of the most commonly-used computer vision, natural language, and audio datasets, and subsequently study the potential for these label errors to affect benchmark results. Errors in test sets are numerous and widespread: we estimate an average of 3.4% errors across the 10 datasets, where for example 2916 label errors comprise 6% of the ImageNet validation set,” say the researchers in the paper titled ‘Pervasive Label Errors in Test Sets Destabilize Machine Learning Benchmarks’. The errors that are very apparent include an image of a baby being identified as a nipple, a photo of a ready to eat pizza being labeled as dough, a whale being labeled as a great white shark and swimsuits being identified as bra.
The problem with incorrectly labeled data sets in machine learning systems is that AI then learns the incorrect identification and knowledge, which will make it harder for AI based systems to deliver the correct results. Or for us humans to be able to trust it at all. AI is now an integral part of a lot of things we interface with on a daily basis, such as web services, smartphones, smart speakers and more. Researchers say that lower capacity models may be practically more useful than higher capacity models in real-world datasets with high proportions of erroneously labeled data. They give the example of ImageNet with corrected data, where “ResNet-18 outperforms ResNet50 if the prevalence of originally mislabeled test examples increases by just 6%. On CIFAR-10 with corrected labels: VGG-11 outperforms VGG-19 if the prevalence of originally mislabeled test examples increases by just 5%.”
Read all the Latest News, Breaking News and Coronavirus News here
Comments
0 comment